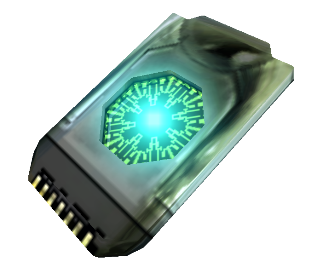
BOSTON: MIT scientists, including one of Indian-origin, have developed a new highly efficient chip that may enable mobile devices to run powerful artificial intelligence algorithms, and help usher in the “Internet of things”.
Neural networks, large virtual networks of simple information-processing units, which are loosely modelled on the anatomy of the human brain are typically implemented using graphics processing units (GPUs), special-purpose graphics chips found in all computing devices with screens.
A mobile GPU, of the type found in a cell phone, might have almost 200 cores, or processing units, making it well suited to simulating a network of distributed processors.
Researchers at Massachusetts Institute of Technology (MIT), including Tushar Krishna, designed a new chip specifically to implement neural networks.
It is 10 times as efficient as a mobile GPU, so it could enable mobile devices to run powerful artificial-intelligence (AI) or ‘deep learning’ algorithms locally, rather than uploading data to the internet for processing.
“Deep learning is useful for many applications, such as object recognition, speech, face detection,” said Vivienne Sze from MIT.
“You can imagine that if you can bring that functionality to your cell phone or embedded devices, you could still operate even if you don’t have a Wi-Fi connection,” Sze said.
The new chip, called ‘Eyeriss’, could also help usher in the “Internet of things” — the idea that vehicles, appliances, civil-engineering structures, manufacturing equipment, and even livestock would have sensors that report information directly to networked servers, aiding with maintenance and task coordination.
With powerful AI algorithms on board, networked devices could make important decisions locally, entrusting only their conclusions, rather than raw personal data, to the internet.
The chip has 168 cores, roughly as many as a mobile GPU has.
The key to Eyeriss’s efficiency is to minimise the frequency with which cores need to exchange data with distant memory banks, an operation that consumes a good deal of time and energy.
Whereas many of the cores in a GPU share a single, large memory bank, each of the Eyeriss cores has its own memory. Moreover, the chip has a circuit that compresses data before sending it to individual cores.
Each core is also able to communicate directly with its immediate neighbours, so that if they need to share data, they do not have to route it through main memory.
The final key to the chip’s efficiency is special-purpose circuitry that allocates tasks across cores.
The MIT researchers used Eyeriss to implement a neural network that performs an image-recognition task, the first time that a state-of-the-art neural network has been demonstrated on a custom chip.